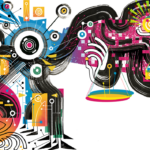
The AI apocalypse is coming. Or it isn’t. Depending on what you read, you might get confused.
One thing is certain: Humans are fired up about smart machines. Much of the attention has focused on ChatGPT, an “artificial intelligence language model designed to generate human-like responses to natural language prompts” (in its own words).
ChatGPT gets coy if you ask whether its existence should be cause for human concern. “It’s important to recognize that I am a tool and not inherently good or bad. It’s how people choose to use me that can have positive or negative consequences,” it says.
Many researchers, however, are not so noncommittal. They see inherent flaws in the machine learning technology that forms the foundation of tools such as ChatGPT, and they would like to make it better.
While ChatGPT advises that “it’s always a good idea to double-check any important information I provide,” some UMBC researchers are working to build better safeguards into the AI systems themselves—AI the public can trust.
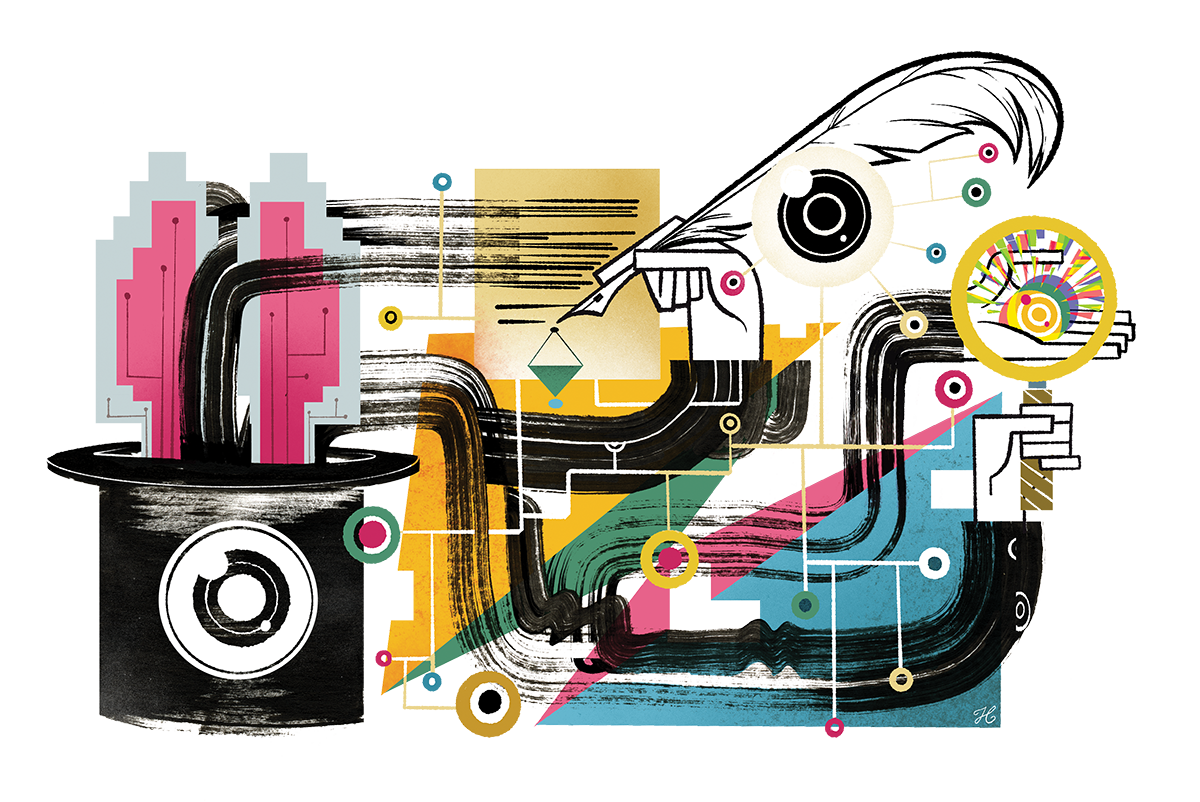
On March 22 of this year, a group including prominent artificial intelligence researchers and tech entrepreneurs released an open letter calling for a six-month pause on the training of powerful AI systems.
“AI systems with human-competitive intelligence can pose profound risks to society and humanity,” the letter argued. “Powerful AI systems should be developed only once we are confident that their effects will be positive and their risks will be manageable.”
The letter signers, including two UMBC faculty, expressed alarm at an AI arms race unleashed with the November 2022 public debut of ChatGPT, a celebrity chatbot that answers almost any question or prompt with humanlike ease. In a mere two months, the bot attracted 100 million users, and big tech companies began sprinting to deploy similar technology in their products.
Yet a general unease is accompanying this latest rush for AI gold.
ChatGPT can dazzle users with its eloquent prose (and poetry!), but it sometimes delivers complete falsehoods. People fret that such technology will eliminate jobs and empower scammers and dictators. And beneath it all, many researchers worry that we do not fully understand—nor can we reliably control—how creations such as ChatGPT work.
“At the core of many powerful AI systems today are what are called ‘blackbox’ models,” says Manas Gaur, an assistant professor in the Department of Computer Science and Electrical Engineering (CSEE) at UMBC. The models percolate data through layers of calculations so dense and complex that researchers struggle to track what’s happening inside. The models may excel at certain tasks—like writing sentences in ChatGPT’s case—but they cannot explain why they make the decisions they do. Sometimes they do perplexing, and erratic, things.
“Some people see ChatGPT and similar technology as a progressive tool while others fear it is dangerous,” says Nancy Tyagi, a master’s student in computer science at UMBC who is also working as a researcher in Gaur’s lab. “In my opinion, such tools are inherently risky and need further analysis. If these models are to be used in sensitive areas such as mental health or defense systems, then more work is required to make them safe, controllable, and trustworthy.”
Tyagi is working on a project to build an AI mental health assistant capable of initiating safe and appropriate conversations based on clinical guidelines in mental health. Her project is one of many that Gaur and other AI researchers at UMBC are launching with the aim of ensuring AI tools are accurate, transparent, and safe.
To better understand these researchers’ quest for trustworthy AI, it helps to take a step back and consider how the latest AI trend fits into the big picture.

A Brief History of
Thinking Machines
When the field of artificial intelligence launched in the 1950s, computers were feeble compared to the muscular monsters that power systems such as ChatGPT today. Yet researchers were intrigued by the possibility of teaching them to think like humans. What followed was a roller coaster of booms and busts.
“The history of AI has been marked by periods of hype, followed by some level of disillusionment,” says Tim Finin, CSEE professor and a researcher at UMBC who has been studying AI problems for more than 50 years.
Driving the ups and downs were three interrelated factors: the power (and limits) of the hardware that formed computers’ brains, the data available to train those brains, and the “thinking strategies” AI researchers devised.
In the beginning, researchers taught machines to play games, learn language, and solve mathematical puzzles using a variety of “thinking” approaches. Yet the field hit a wall in the 1970s: Computers couldn’t store enough information or process it fast enough to tackle real-world problems. This was the first “AI winter,” when funding dried up and the topic faded from public view.
The birth of the microprocessor at the end of the decade revitalized AI research. Riding the shoots of this new life, a certain approach to machine thinking rose to prominence—that of the expert system. These AI programs were based on pre-programmed knowledge and logic meant to mirror the reasoning of human experts. Perhaps the most famous expert system was IBM’s Deep Blue, which beat the Russian chess champion Garry Kasparov in a chess match in 1997.
Expert systems could shine when solving narrowly defined problems (such as winning a game of chess), but they were brittle, says Finin. The systems struggled to adapt to fuzzy and fluid real-world situations, and it was cumbersome to program all the rules that an expert might use to evaluate a problem.
As the limits of expert systems became clear in the 1990s, AI felt the chill of a second winter.
It was another advance in computing hardware that thawed the field again after the turn of the 21st century. The graphic processing units developed to enhance video games supercharged computers’ speed and power at low cost. This, coupled with a flood of free data from the internet, propelled a new type of AI to the forefront: machine learning.
With loads of computing power and heaps of examples to learn from, researchers found surprising success getting computers to teach themselves how to think. The computers start with a question, perform some calculations, and guess the answer. They then compare it to the actual answer. If they are wrong, (which they usually are at first), they fiddle with the calculations and try again. After running billions of calculations, such systems can become quite proficient at tasks such as identifying images of cats and predicting the next word in a sentence.
THE SEASONS OF AI
The growth of the modern field of AI has been marked by a series of rapid spurts, followed by more dormant periods. People often liken these ups and downs to the seasons. During AI summers, public attention shines hot on the field. Yet the bountiful fruit of the season has often grown from seeds of ideas planted during quiet AI winters.
Summer 1: Expert systems
AI programs based on knowledge and logic flourished in the 1980s. Examples include systems that can identify unknown chemicals, diagnose diseases, and play chess. The systems are safe and explainable, but fail to adapt to fluid and complex situations.
Summer 2: Machine learning
Starting in the early 2010s, the potent combination of supercharged computing and heaps of free internet data powered AI’s second summer: the golden era of machine learning—an era that we are arguably still in.
AI systems started to recognize images, transcribe and translate language, and create text and art almost like humans do. These systems have surprised even their own creators with their range of abilities, but they are hard to understand, reason with, and control.
Into the future: Hybrid AI
It’s not yet clear when or if the second AI summer will turn to fall. But researchers are already planting the seeds for future advances. Combining the fruits of past summers, researchers hope to make future AI systems that are adaptable and safe, self-taught and able to explain their decisions.
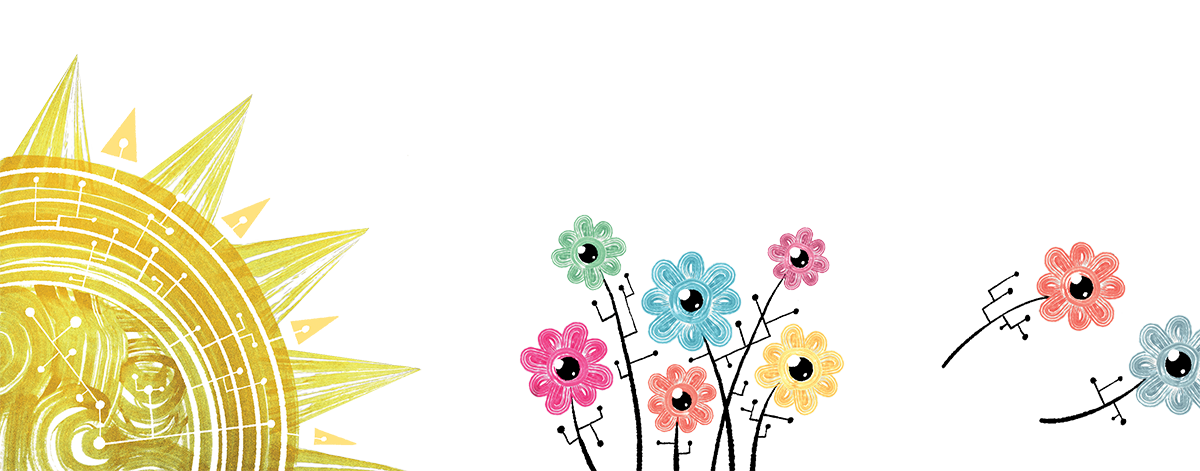
FUN FACT:
UMBC’s first Ph.D. graduate in computer science, Sanjeev Bhushan Ahuja, earned his degree when expert systems dominated AI. His dissertation, published in 1985 and titled “An Artificial Intelligence Environment for the Analysis and Classification of Errors in Discrete Sequential Processes,” advances techniques popular during this time.
This approach to machine learning is called a neural network, so named because it was originally inspired by the way neurons in the brain work. Neural networks lie at the heart of most famous AI applications today, including image classification tools, voice recognition, and text and image generators.

The power (and limits) of
machine learning
When many of the new machine learning systems debuted, their powers seemed almost miraculous. But soon enough, drawbacks emerged. The machines require enormous data sets (and enormous amounts of energy) to learn. They will adopt biases from their training data and sometimes from their interactions with humans. A chatbot named Tay was quickly scuppered after its 2016 release, when users pushed it into spewing racist and sexist ideas.
Machine learning systems can also fail spectacularly in individual instances (even if they get answers correct most of the time). For example, a driver was killed in 2016 when the autopilot in a Tesla car failed to recognize the side of a white trailer truck against a bright sky.
The blackbox nature of state-of-the-art machine learning means the systems are unable to explain or justify their conclusions, giving users—and even their own creators—little insight into their thinking. For the most part, the systems struggle to build consistent worldviews or reason logically.
The weaknesses of learning models also leave them susceptible to malicious manipulation. Adversaries might “poison” the data used to train the models or exploit the model’s opaqueness to hide an attack.
“It is time we fall back from trusting these models,” says Gaur, whose personal push to make AI systems more explainable, robust, and safe is part of a growing international movement.
Another UMBC researcher joining the push is Houbing Song, a professor in the Department of Information Systems at UMBC. Song says that transportation, defense, medicine, and the law are some areas where explainable and safe AI systems are needed the most.
As researchers tackle the challenge of making current AI systems better, they are often returning to ideas from an earlier era of AI.
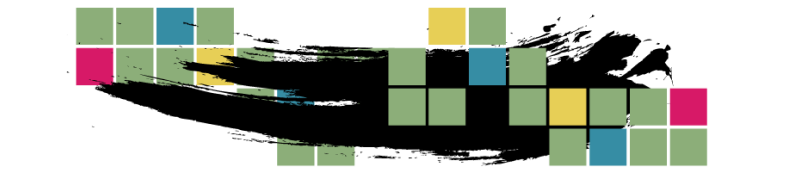
Hybrid systems to merge logic and learning
If the AI systems of the 1980s married the AI systems of the 2010s, their baby might be the type of system Gaur, Song, and others are working to develop.
These systems look to deliver the learning capabilities of neural networks alongside the safeguards of knowledge and rule-based systems.
In the field of mental health, Gaur points out that current chatbot systems are not well suited to answering patients’ questions since they can give unsafe or off-the-wall responses.
“Guaranteeing these systems’ safety calls for more than just improving their overall performance” he says. “We must also make sure the systems are prevented from giving risky answers.”
Working with Karen Chen, an assistant professor from the Department of Information Systems, Gaur has written a paper highlighting the properties that AI-powered virtual mental health assistants should exhibit to be considered safe and effective.
Creating “AI Scientists” at UMBC
New scientific discoveries often lay the groundwork for significant advances in human well being. Think of medical treatments that spring from a better understanding of the human body or labor-saving devices we fashion using our knowledge of material properties.
Tyler Josephson, an assistant professor in the Department of Chemical, Biochemical, and Environmental Engineering, hopes to turbocharge science’s discovery engine, with a little help from AI.
Josephson has started a new project to translate chemical theories into a machine-readable mathematical language. Once the computers have access to the foundations of science, Josephson believes they could be tasked with logically manipulating that information to reveal new discoveries.
You might wonder if Josephson has any worries about creating his own AI-powered replacement. But he doesn’t think AI scientists will displace the human kind.
“I think scientists have so many different problems to solve. And if we solve them faster with AI, they just open up brand new questions for us to go after next,” Josephson said in an interview about his work with the Canadian radio program Quirks & Quarks.
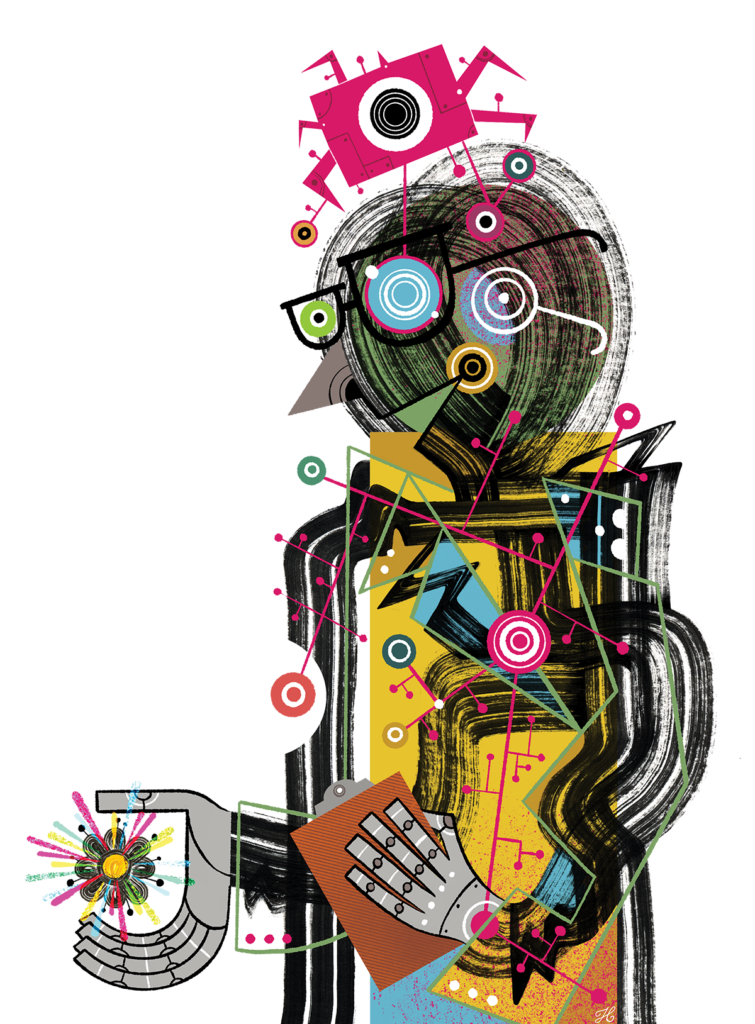
Together with his students, he is also working to create such systems. Using an approach called knowledge-infused learning, the researchers are looking to anchor their AI systems in clinically approved guidelines. They are also pushing their systems to reveal their thinking so that the approaches can be checked by mental health experts. Sometimes the results reveal that even when a system arrives at a correct conclusion, the information it used to reach that conclusion may be irrelevant to a human doctor’s thinking.
Song has also been coaxing AI learning models to open up. In a recent paper, he and his co-authors developed a tool to identify attacks on an image-recognition program by figuring out which parts of its neural network are most susceptible to manipulation.
In the fall of 2023, he will be teaching a new graduate-level course on a broad category of hybrid AI called neurosymbolic AI. UMBC will be only the second university in the world to offer such a course, he says.
Song arrived at UMBC in January on the heels of winning major honors for his research in computing and engineering and is looking forward to turning more of his attention to this emerging frontier in AI research. He says he eventually hopes to build a world-class AI research institute at his new academic home, focused on delivering learning machines that can be confidently used when safety is a top priority.
“I recognize the need for trustworthy AI,” Song says. “I believe that this field of research is where I can make unique contributions and take on responsibilities for my professional communities and my home institution.”

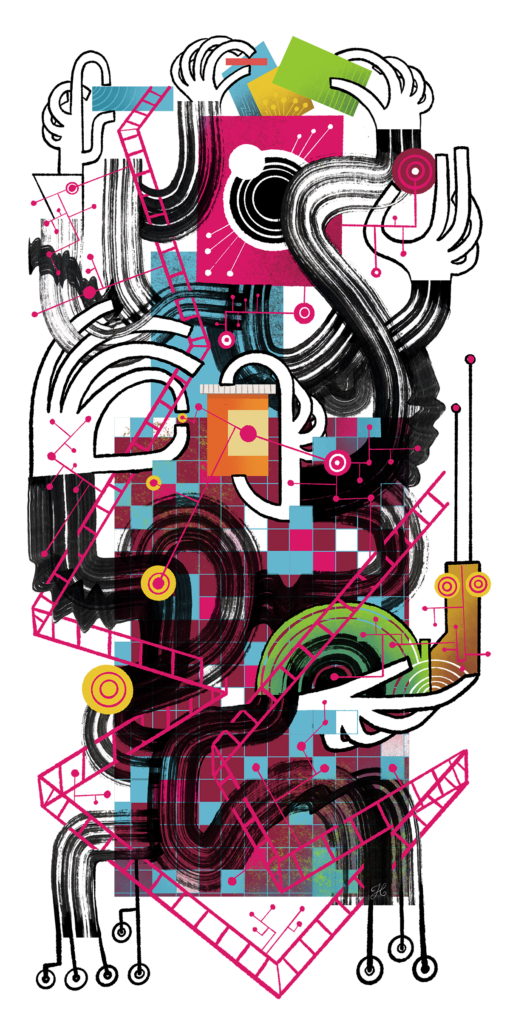
Technology to benefit society
The initial goal of AI, as defined by a group of researchers credited with launching the field at a 1956 workshop, was to “make machines use language, form abstractions and concepts, solve [the] kinds of problems now reserved for humans, and improve themselves.” But if the aim is human-like thinking, it naturally raises the question: How do humans think?
In a bestselling book titled “Thinking, Fast and Slow,” world-renowned psychologist Daniel Kahneman posits that humans have two thinking systems: a fast one and a slow one. The fast one is the thinking that comes to mind almost without effort, and we use this thinking most of the time. Yet it is prone to errors. The deliberative slow thinking system catches mistakes and enables breakthroughs in understanding.
Finin compares machine learning models to the fast-thinking system while knowledge and logic-based systems are more like the slow-thinking system. To make today’s faddish, fast-thinking models more competent, researchers such as Gaur, Song, and their students are extending them with slow-thinking capabilities.
We may still be decades away from AI systems approaching the full range of human intelligence. There are many ethical questions to grapple with before we reach a Hollywood-esque future of self-flying cars and android coworkers. Yet the decades of AI research up to this point have already transformed the world. AI concepts underpin the ways we search the web, shop online, and otherwise interact with the digital world.
AI has enormous potential to improve human lives, but we must proceed wisely. UMBC researchers are at the frontiers of AI research, pushing the limits of knowledge and theory, and striving to make the technology better for the benefit of society.
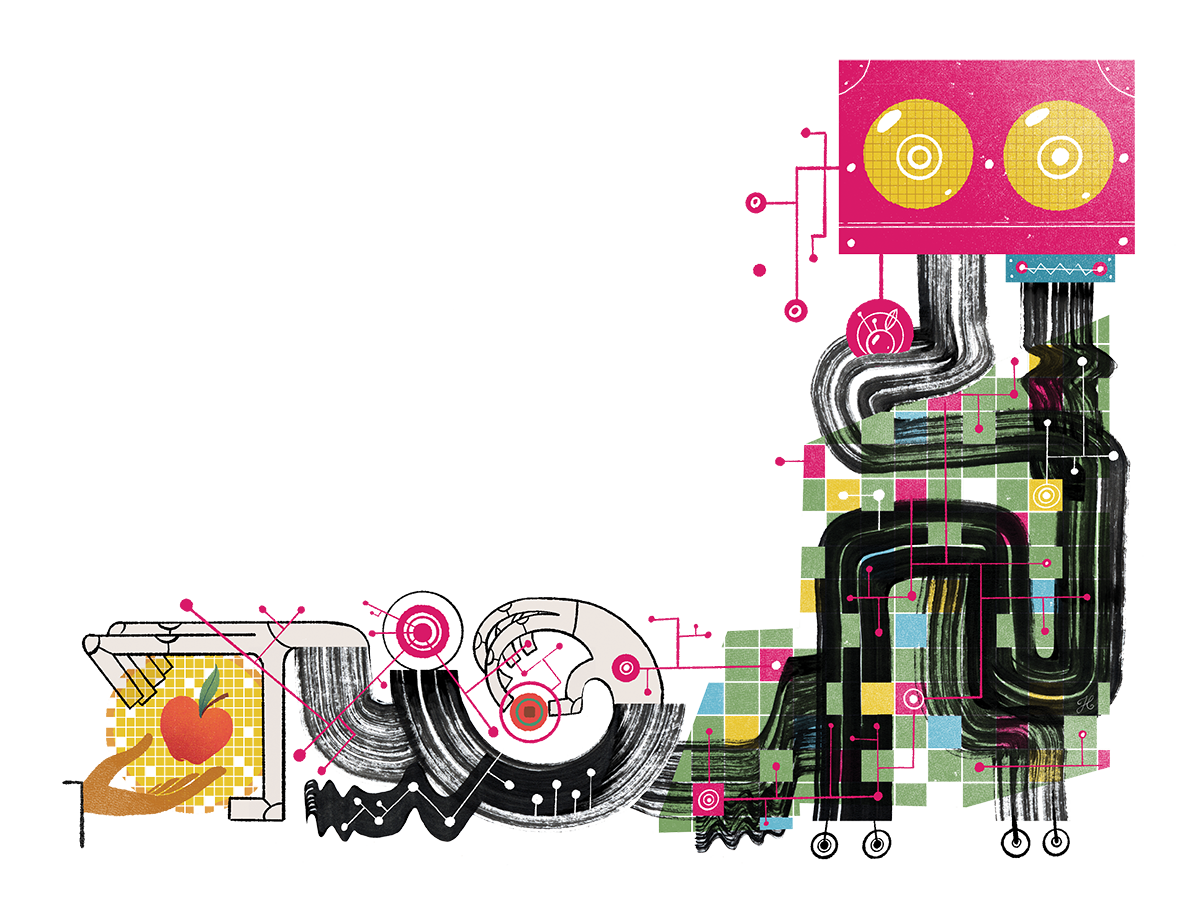
Training Your Robot Assistants
If you hope the AI revolution will bestow humanity with machine “Jeeves” capable of meeting your every need, Cynthia Matuszek has some bad news. “I’m always being asked: ‘When will we have robot butlers?’ I have to say—not any time soon,” says Matuszek, an associate professor in the Department of Computer Science and Electrical Engineering.
Matuszek researches how to build robots that understand human commands in complex and chaotic natural environments. She has successfully trained a robot hand that can respond to written prompts such as “Grab the apple.” She is also exploring how to teach robots to understand spoken language and to learn new concepts, such as how to dice a vegetable, if a human shows them how.
Part of what motivates Matuszek’s work is the huge unmet demand for caregivers to assist people as they age. Robots might fill the gap. Matuszek says we likely won’t have “Jack of all trades” helpers, but robots could specialize in certain tasks, such as preparing food or folding laundry.
Another part of what motivates Matuszek is the thrill of being the first person to discover how to do something new. “It’s really just so much fun,” she says.